视频图像语义标注方法研究
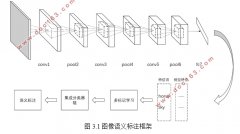
1.无需注册登录,支付后按照提示操作即可获取该资料.
2.资料以网页介绍的为准,下载后不会有水印.资料仅供学习参考之用.
密 惠 保
视频图像语义标注方法研究(任务书,开题报告,论文12000字)
摘 要
随着互联网、多媒体技术、存储技术等的飞速发展,以及各种数字设备普及,使得海量的多媒体数据得以快速的增长和传播。如今数字图像已经与我们紧密的联系在一起,比如新闻媒体、医疗行业、娱乐行业、工业制造业等,在被如此广泛应用的背景下,图像数据的数量在以指数的形式增长,然而面对如此庞大的图像数据,如何进行有效的分类和检索已经成为迫切需要解决的难题。
本文主要从生成式的图像特征提取和判别式的图像语义学习两个方面来展开研究的,通过结合卷积神经网络和SVM构建了一个基于生成式特征提取和判别式语义学习的图像语义标注框架。首先用标注好的图像数据集训练卷积神经网络,将训练好的网络作为图像的高层特征提取器,再利用图像提取到的高层特征对SVM进行训练,从而将图像的标注问题转化为多标签分类问题,进而完成图像的语义标注。对比一些传统的图像语义标注方法,该方法取得了较好的标注效果,所得结果对于图像自动语义标注具有重要的指导意义。
关键词:深度学习;SVM;图像语义;语义标注
Abstract
With the rapid development of the Internet, multimedia technologies, and storage technologies, as well as the popularity of various digital devices, the rapid growth and spread of massive multimedia data has been achieved. Nowadays, digital images have been closely linked with us, such as news media, medical industry, entertainment industry, industrial manufacturing, etc. Under the background of such a wide range of applications, the number of image data has grown exponentially, but With such huge image data, how to effectively classify and retrieve has become an urgent problem to be solved.
This dissertation mainly focuses on two aspects: the generation of image feature extraction and the discriminative image semantics learning. An image semantic annotation framework based on generated feature extraction and discriminative semantic learning is constructed by combining convolutional neural network and SVM. . Firstly, the convolutional neural network is trained with the labeled image dataset. The trained network is used as the high-level feature extractor of the image, and then the SVM is trained by using the high-level features extracted from the image, so that the labeling problem of the image is converted into multiple labels. Classification problem, and then complete the semantic annotation of the image. Compared with some traditional image semantic annotation methods, this method has achieved better annotation results. The results obtained have important guiding significance for automatic semantic annotation of images.
Key Words:Deep learning;SVM;Image semantics; Semantic annotation
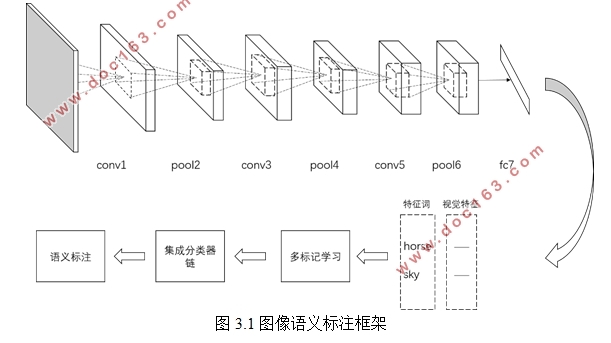
目录
摘 要 I
Abstract II
第1章 绪论 2
1.1 研究背景及意义 2
1.2 国内外研究现状 3
1.2.1图像标注模型的研究 3
1.2.2 解决语义鸿沟的现有方法 4
1.2.3 传统的图像特征提取 4
1.3 主要研究内容和章节安排 4
第2章 图像语义标注方法介绍 6
2.1 基于传统算法的图像语义标注 6
2.1.1 基于分类的图像语义标注方法 6
2.1.2 基于概率统计模型的图像语义标注方法 7
2.1.3 基于关联文本的图像语义标注方法 8
2.2 基于深度学习的图像语义标注 8
2.3 本章小结 9 [来源:http://www.think58.com]
第3章 融合深度学习和SVM的图像语义标注 11
3.1 图像的语义标注 11
3.2 基于卷积神经网络的图像特征学习 11
3.3 基于SVM的图像语义学习 13
3.4 本章小结 14
第4章 图像语义标注算法实现 16
4.1 数据集与实验设置 16
4.2 实验结果与分析 17
4.3 本章小结 18
第5章 总结与展望 19
5.1 论文工作总结 19
5.2 研究工作展望 19
参考文献 21
致 谢 23 [资料来源:THINK58.com]