基于卷积神经网络的物体检测与分类
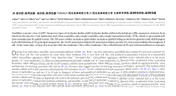
1.无需注册登录,支付后按照提示操作即可获取该资料.
2.资料以网页介绍的为准,下载后不会有水印.资料仅供学习参考之用.
密 惠 保
基于卷积神经网络的物体检测与分类(任务书,开题报告,外文翻译,论文说明书18000字)
摘 要
在计算机视觉领域,目标检测可以说是一个非常重要的研究方向,随着它的逐渐发展改进,在如今也是非常火热的研究领域,数以万计的研究员都在朝着这个方向发力。在视频或图像中检测出特定的目标并加以标记是目标检测技术的最终任务,对于这种任务的需求,也是随着社会的迅速发展而产生的,国家越来越富强,人们对生活的质量也提出了更高的要求,为了省出更多的时间做高素质要求的工作,我们需要把更多的机械性工作交由机器来完成,因此机器学习才发展起来并在许多领域做出了不小的贡献,包括国防安全、资金维护、人机交互、模式识别等,正是由于取得良好的效果,国家人民对它的需求也越来越大,硬件方面的发展也保证了它会有光明的前景,所以研究目标检测可以说是具有非常重要的意义,深度学习的出现刚好适应了时代的发展,其中表现极好的卷积神经网络凭借自身结构设计上的优点,在目标检测的发展中画上了浓重的一笔。
本论文对目标检测算法这个课题进行了研究,主要的研究工作包括:(1)先介绍了目标检测算法的发展背景及发展现状,以及在本篇文章,研究它的意义所在。(2)分析了目标检测的一些基本理论知识,还有一些重要技术应用。(3)简单介绍了一下近些年来目标检测算法的一些关键成果的核心思想及实际效果对比。(4)重点研究Faster R-CNN算法的基本原理框架,分析其在性能上与以往算法的提升。(5)在PASCAL VOC2007数据集上,基于Faster R-CNN的算法,利用深度学习tensorflow的框架训练得到了比较理想的模型,能对用户输入图片处理得到比较精确的判别结果。
[来源:http://think58.com]
关键词:目标检测;深度学习;卷积神经网络;图像识别
Abstract
In the field of computer vision, target detection can be said to be a very important research direction. With its improvement, many researchers are working in this direction in today's very hot research field. Detecting and marking specific targets in video or images is the ultimate task of target detection technology. the demand for this task is also produced with the rapid development of society, and the country is becoming stronger. People also put forward higher requirements for the quality of life. In order to save more time to do the high quality work, we need to hand over more mechanical work to the machine to complete. Therefore, machine learning has made great contributions in many fields, including national defense security, capital maintenance, human-computer interaction, pattern recognition and so on. It is precisely because of the good results that the people of the country need it more. The development of hardware also ensures that it will have a bright prospect, so the research of target detection can be said to be of great significance. The emergence of deep learning have just adapted to the development of the times, in which convolution neural network, which has excellent performance because of the advantages of its own structural design, has drawn a heavy stroke in the development of target detection.
In this paper, the target detection algorithm is studied. The main research work includes: (1) the development background and present situation of target detection algorithm are introduced, and the significance of our research on it is also introduced.(2) some basic theoretical knowledge of target detection and some important technical applications are analyzed. (3) the core idea and practical effect comparison of some key results of target detection algorithm in recent years are briefly introduced.(4) the basic principle framework of Faster R-CNN algorithm is studied, and the improvement of its performance compared with the previous algorithms is analyzed.(5) on the PASCAL VOC2007 dataset, based on the Faster R-CNN algorithm, the ideal model is obtained by using the framework training of deep learning tensorflow, and the more accurate discriminant results can be obtained for the user input picture processing.
Key Words:Target detection; depth learning; convolution neural network; image recognition.
[来源:http://www.think58.com]
本文的主要研究内容
本论文研究了目标检测与分类的一些基本知识,关键性技术及它的发展后,选用了在原始基础上做了许多改进,并已被测试出不错性能的基于Faster R-CNN算法的目标检测的网络模型,具体的研究工作如下:
首先,我们对基于卷积神经网络的目标检测与分类的发展境况简单地分析和陈述总结,并对目标检测的一些最最基础的大体结构,每个结构负责什么任务进行了有条不紊的介绍。
接着,我们介绍了关于目标检测的最为关键的结构基础-卷积神经网络,介绍了它的基本组成以及它是如何工作的,以及它们用到的一些关键性算法的原理。
然后,我们先介绍了其他相关算法包括R-CNN、Fast R-CNN,在此基础上分析了基于Faster R-CNN算法的卷积神经网络在原有基础上的进步在哪些方面,做了这些改进后,网络得到了对应的哪些优势。
实验部分,我选用了使用 pascal voc2007 数据库对我们基于Faster R-CNN算法而搭建的神经网络架构进行了训练与测试,并用统计评估模块对,测试的结果进行了数据指标上的评价,包含了精确度和效率指标,挑选了一些图片结果进行效果分析评估,最后输入实际生活中的图片进行可行性验证得到了良好的处理结果。
最终,借效果不太好的地方,分析原因提出可能有效的改进方案,对目标检测整个进步大方向的做了个人预测,发表了一些看法。 [来源:http://www.think58.com]
1.4本论文的结构安排
第一章,我先介绍了本论文中我研究目标检测与分类这一课题的意义是什么,并介绍了这一课题的发展如何,比较流行的有好的效果的技术有哪些,挑选一些关键简要的讲解一下核心设计方法,接着,详细地陈列了我所做的主要研究工作,然后总结了本文的结构框架。
介绍了目标检测的基本理论知识包含最基本的大体框架模块设计,最基本的卷积神经网络的发展以及它是如何模拟人的神经元的,这样的结构下用什么样的算法来训练出理想化的权重。
介绍Faster R-CNN的结构和原理,我们先介绍了R-CNN和Fast R-CNN的网络框架和性能分析才引出Faster R-CNN,分版块讲述了它的特征提取模块和候选框生成模块以及分类回归模块,损失函数的设定,最后还加了网络性能的一些评价指标。
这一部分是实践部分,讲解了我的具体操作细节,具体包含了我所选用的数据集介绍,我是在什么环境下运行程序的,训练时一些关键参数的设定,测试选用的数据集,最后是测试结果展示分析和统计计算下的性能评估。
这是整个文章的总结部分,这里我总结了我在整个过程到底做了什么,做出来的步骤是什么,做出来的东西的效果又如何,再反思一下,我需要对我做的东西进行具体哪些方面的优化的,也就是对整个课题的总结和反思。
[资料来源:http://www.THINK58.com]
目录
第1章 绪论 1
1.1 研究背景及意义 1
1.2 国内外研究现状 2
1.3本文的主要研究内容 3
1.4本论文的结构安排 4
第2章 卷积神经网络与目标检测概述 5
2.1 神经元 5
2.2神经网络 7
2.3 反向传播算法与训练 8
2.4卷积神经网络 9
第3章 基于Faster R-CNN算法的目标检测 11
3.1网络结构 11
3.1.1 R-CNN 11
3.1.2 Fast R-CNN 11
3.1.2 Faster R-CNN 12
3.2特征提取网络 13
3.3生成候选框RPN 14
3.4分类回归网络 16
3.5评价指标 17
第4章 实验步骤及结果分析 20
4.1数据集准备 20
4.2实验环境参数配置及训练 22 [来源:http://think58.com]
4.3模型评估 23
4.4实验结果展示与分析 26
4.5 demo演示 28
第5章 总结与展望 30
5.1主要工作总结 30
5.2目标检测算法的发展与展望 30
参考文献 32
致 谢 34 [版权所有:http://think58.com]